Discretionary Managers Seek Alpha in Alternative Data
May 30, 2017 | By: Ivy Schmerken
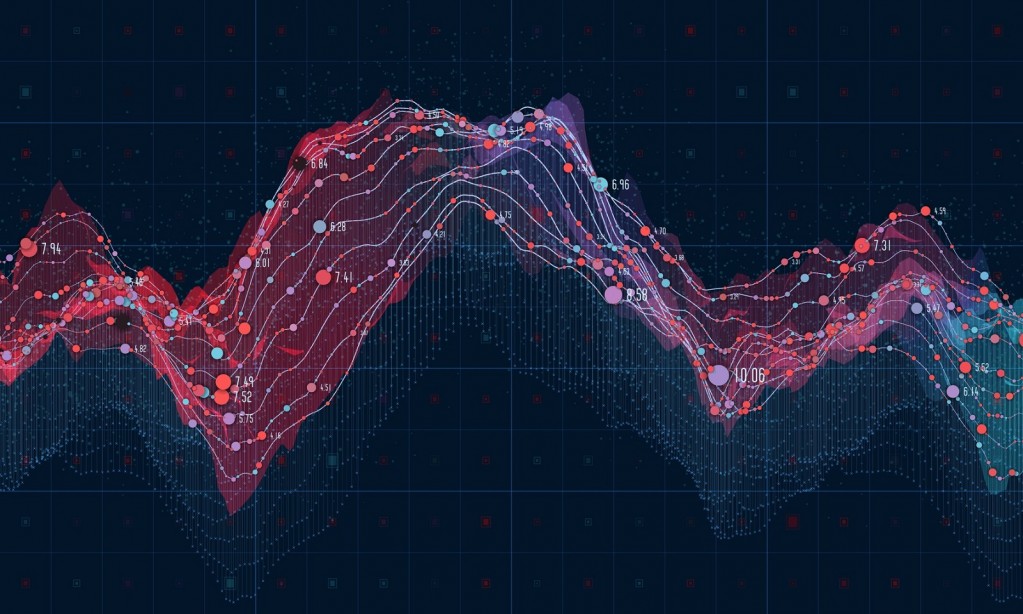
By Ivy Schmerken, Editorial Director
Alternative data providers see huge potential in providing their data to discretionary asset managers who are losing assets to quantitative and systematic funds.
As active managers trail the performance of passive index funds and exchange-traded funds (ETFs), discretionary fund managers are scrambling to consume big data analytics into their decision making process.
While early movers in the big data analytics industry have mainly been quant hedge funds and systematic fund managers, the next wave is going to be discretionary fund managers, according to panelists at an event sponsored by Wall Street Horizon, Estimize, OTAS Technologies and FlexTrade Systems.
“We’re at the point of crossing the chasm. The early adopters have adopted it. And [now we’re] crossing that barrier between early adopters and the mainstream,” said Vinesh Jha, founder and CEO at ExtractAlpha, who spoke at “Uncovering Alpha in Alternative Data Sets” held on May 17.
There’s been an explosion of alternative data sources, such as satellite imagery data and credit card data that didn’t exist 10 years ago, said Leigh Drogen, founder and CEO of Estimize, an open earnings estimates platform, speaking at the event.
However, discretionary asset managers have more difficulty incorporating big data sets because they lack a process, said several panelists.
“The challenge is to provide this content into the trader workflow of the discretionary managers who are not even quants who have a hypothesis about how the market works,” said Tom Doris, founder and CEO at OTAS. Technologies, on the panel. “They look at those signals and data sources in an ad hoc fashion,” said Doris.
Quantitative hedge funds such as Bridgewater and Renaissance Technologies have invested in big data analytics and generated higher returns than many of their peers. “Everybody wants to be them because they want to have returns like them in the systematic quant space,” said Jamie Benincasa, senior vice president and head of global sales at FlexTrade. These firms are household names in the hedge fund space that are probably on an 8-10 year run.
But these firms are not going to reveal which data sets are of value, “or precisely tell you how it works,” said Benincasa. “They want as much data as they can get and they will spend the money to do so,” he said.
Pointing to Quantopian, a platform for quants to create and test their own algorithms, Benincasa predicts that the next generation of alpha generation is going to come from that crowd. Anyone can open an account on Quantopian’s network and create its own algorithms. Quantopian then monitors performance and allocates capital to quants with the best performing strategies.
Defining Alternative Data
While big data is a nebulous term, perceptions are also shifting on what constitutes alternative data. “There has been a bunch of data sets sitting out there but no one thought of them as data,” said Jha. News analytics and social media analytics companies are turning text from 10Qs and 10K filings and earning call transcriptions into data that computers understand. That means turning this data into sentiment or extracting data from them. “Fundamental investors have been using this data for years, but this is alternative data for quants,” said Jha.
Even some of the older data such as earnings estimates has been optimized and is now perceived as alt data. “Our data is the quintessential example of an old data set, an earnings calendar,” said Barry Star, Wall Street Horizon’s founder and CEO. ”We reinvented it,” said Star. “Individually the events that we track could be considered old alpha, but people are constantly coming up with new ways to utilize the data, “ he said. Take the case of Date Breaks— if the earnings date for a company moves from Monday to Thursday there’s all kinds of information involved in how many days the date moved. Does it move forward? Does it move backward? There is alpha all over that information,” Star said.
Challenges with Workflow Integration
But it can be challenging for hedge funds and asset managers to integrate big data into their front-end investment and trading processes.
Drogen said he’s been contacted by discretionary funds that want to build a quantitative process. Someone with “a good quantitative background in a discretionary firm takes our data, reads our research and takes our factor model and figures out if it would have added alpha.” But the decision is left to the portfolio managers. “The PMs have to buy into it. It is religion vs. a science problem. If they don’t solve this, they’re going to get left behind,” insists Drogen.
According to Doris, the difference between systematic and discretionary manager is mainly that one is process driven, and the other is non-process driven. “So-called systematic managers tweak their models more than discretionary firms alter their portfolio holdings,” he argued. “If they’re already systematic about their approach, they can use the data. It’s not onerous for them to change their process.” The problem then is to get the big data and analytics into the trader’s workflow, said Doris.
“Quants will take the signals directly into a black box, then push out trades via an API to the market because they don’t want anybody to see them,” said FlexTrade’s Benincasa. However, “discretionary managers are looking to funnel the data through their order and execution management systems.”
To bring alternative data into the trader’s workflow, Benincasa cites the integration of the FlexTRADER EMS with OTAS’s market analytics delivered with natural language processing technology, and the Symphony messaging system. From OTAS, traders can capture the natural language, highlight it and send it via Symphony to the PMs. Then the PMs can send an order back, and the trader highlights the order, clicks, and the order lands on FlexTRADER’s blotter. Additionally, social media is available as heat maps and ticks on the blotter, so a trader can now run an alert saying a particular name spiked, get social media sentiment in this name, and determine what is going on, he said. “We’re in the business of consuming these analytics and providing traders with the ability to feed it back from the market to the PMs who may not have access to these data sets,” said Benincasa.
Despite some of these hurdles, discretionary firms are striving to harness alternative forms of data. “The main variable is how they build their teams,” said Drogen. Some firms make the mistake of building a data science/quantitative research team in a separate building and then selling Excel spreadsheets and research reports to the PMs. Those that have done it right build a trading desk with a quant, two engineers, a data analyst, the PM and fundamental analysts sitting together, suggests Drogen. Then there’s a data acquisition and repository team on top of that responsible for buying data and other things. “The PM needs to operate as a pod where those quants and engineers understand the process,” said Drogen.